The Book Of Why (2018) Book Summary and Insights
Book Title: The Book Of Why
Subtitle: The New Science of Cause & Effect
Publication Date: 2018
Author Names: Judea Pearl & Dana Mackenzie
Book Summary
This is a book that banishes to the depths of illogical fallacy the age-old adage that “correlation does not imply causation” which has rather become stale in this age of technology- and data-driven results. The authors dispel these notions through the use of math and statistics. The book of why helps us to sift fact from fiction, to understand the concepts of causation and correlation, and the means of applying these concepts to our daily lives. This book enables us to understand some basic and fundamental concepts in our lives and how things are what they are. To find out more, please read the insights below.
Who Is This Book For?
It is for social scientists and every other person who rely on data and statistical information for their daily activities.
About The Authors
Judea Pearl is a `Professor of Computer Science at UCLA. The author of three highly influential scholarly books, he is a winner of the Alan Turing Award, often considered the equivalent of the Nobel Prize for computer science. He is a member of the U.S. National Academy of Sciences, and was one of the first ten inductees into the IEEE Intelligent Systems Hall of Fame. He has received several numerous awards and honorary doctorates, including the Rumelhart Prize (Cognitive Science Society), the Benjamin Franklin Medal (Franklin Institute) and the Lakatos Award (London School of Economics). He is the founder and president of the Daniel Pearl Foundation. He lives in Los Angeles, CA.
Dana Mackenzie is a PhD mathematician turned science writer, and has written for such magazines as Science, New Scientist, Scientific American, Smithsonian, Nautilus, and Discover. His book, The BigSplat, or How Our Moon Came To Be, was named a Booklist Editors’ Choice and selected as an Audiobook of the Year for 2010 by Audible.com. He received the 2012 Communication Award (Joint Policy Board for Mathematics) and the 2015 Chauvenet Prize for mathematical exposition (Mathematical Association of America). He lives in Santa Cruz, CA.
Buy Book: Support The Book Authors And Our Work
Great books should be read, studied and reviewed, so reading the actual book may provide more value to you than the book insights on this page. This will also support the work of the book author and what we do on LarnEdu.
Important Notes
We get a small compensation from Amazon when you visit your nearest/local Amazon site via our affiliate link to purchase an item within 24 hours or if you add it to your cart and checkout within 90 days. This is no additional cost to you and supports our work.
The information on this page is meant to supplement the actual book(it is not a book review but distils the key insights or ideas from the book in under 5000 words). The content creator or LarnEdu does not necessarily support the views, thoughts, and opinions expressed in the text/book. Reasonable skepticism should be applied with any views, thoughts or opinions expressed/shared by the book author or content creator.
Reading the contents of this page does not guarantee specific results. The best lessons are achieved from taking consistent action in the real world rather than being addicted to the illusion of progress by getting stuck on reading an infinite amount of books or book summaries and insights. LarnEdu and the content creator accepts no responsibility or liability for the accuracy of the information on this page or how it is used.
Book Insights
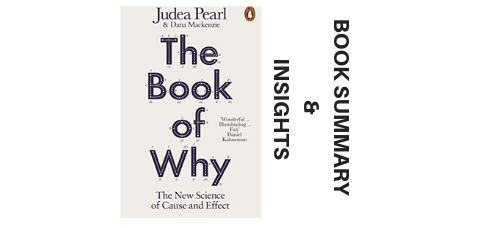
Causation Has For Long Being Discredited By Statisticians
For so long we have heard the mantra correlation is not causation. Correlation means that there is a nexus between a subject and an object. And as the subject changes so does the object. Causes, meanwhile, can get misplaced in a whole haystack of competing issues that a mathematical algorithm could not find it. But there have been situations where Correlation between an object and a subject has been so strong, it has brought about the need to find a cause which has proved correct. For example, the correlation between asbestos and lung disease. The correlation was so significant; it moved scientists to discover causal links. A scientist by the name Wright was able to show causation through correlation using data. But because of the times, they saw him as a joker and ridiculed his research. But with the improvement in technology today, we can see Wright was on the right path. Correlation and causation has helped us understand so many phenomena in our society such as diseases in healthcare and greenhouse gases in climate change.
Data Without Cause Can Be Misleading
Statistical analysis has for a long time, being the pillar of support for Big Business and is today more important than it was yesterday. We use the data from such an analysis to solve problems which hitherto would have been difficult to unravel. Data helps us to make sound decisions and improves our judgment in tricky or uncertain situations. It is no surprise data is valuable in the e-commerce industry. We use data to optimize goods and services to make more sales and target more consumers. Big tech giants like Google and Facebook use data in their services and this is one reason they are some of the biggest advertising companies in the world. But we can manipulate the stats behind these data if we do not take extreme care. Statistics are prone to manipulation. And when this happens, it makes it possible for such statistic to be misleading and result in bad data. It is important now to know what a misleading statistic is or can be. Misleading statistic is the abuse or misuse of a numerical data. The results from such misuse provides poor and misleading information.
Association and Probability: Stage One in the Ladder of Causation
Human beings are hard wired to examine their environment to make connections between subjects and objects. This is an ability many animals possess and is the basis for fundamental machine code. A lion hunting in the savannah looks out for injured or weak prey. By targeting an injured zebra, the lion has statistically increased its chances of having a good meal. But at this lowest level, the lion does not know the why. Why is the zebra injured? Was it because of a previous attack by another lion? Or did the zebra break its foot while jumping? The lion cannot make predictions beyond this level of causality. And this is the reason self-driving technology has not taken off as rapidly as expected. Because self-driving programs cannot see beyond this stage to make accurate predictions beyond the level of association.
Stage Two: Intervention
Intervention unlike association is more active than passive. It is looking at the situation, analysing it, and making a change. It involves making actionable steps to situations to influence the outcome. For example, when we feel hungry, we look for food to eat. Why do we look for food to eat? Because we are hungry. Because we feel hunger pangs which is an unpleasant feeling, we try to influence that unpleasant feeling to produce a pleasant outcome by getting food to eat. But only humans can do this regularly. Machines cannot. We program everything a machine is to do into its code. As a result, it cannot independently solve problems on its own. Every solution a machine or computer can provide, we already calculate in multiple scenarios and feed into its code. But human beings can intervene in situations using controlled experiments. By carrying out such experiments, we can determine the outcome of certain events where a set of principles or actions are applied. And the important thing is, they can learn from such experiments to improve their lives. Intervention is the ability to test an event and bring out a solution and in this stage, it is only man that has the ability to carry out such actions as a normal part of his life. But a few animals with a high level of awareness can do this too. The orca can hunt and bring down prey using complex solutions. While a lion or other big cat will prefer to hunt weak or injured animals to increase its statistical probability of having a meal, an orca or a pod of orcas will find a solution to bring down that strong seal especially where the seal is out of its reach. One such a tactic is the “wave wash” where a pod of orcas build a current of waves to wash out a seal sitting atop an ice floe. But a lion cannot think or apply complex answers to its hunting techniques. If a prey is out of its reach, it will prioritize its strength to hunt another prey.
Final Stage: Imagination
Alas, we get to the final rung where humans inhabit alone. Unlike the previous stage where some select animals can intervene, this stage requires applying complex actions to hypothetical situations. It is the ability to look at non-existing scenarios and find answers to such scenarios. It is not only applicable to hypothetical situations but also to past events. Example of such a situation would be: would my aunt still be alive if she did not have cancer? While humans can answer such questions, machines are incapable of doing so. To build a machine that could do this is akin to having a machine that could predict the future. Like we said before, all machines know how to do is to work with set parameters fed into their programming. Because of this, they cannot independently solve questions on their own talk more of hypothetical scenarios.
The key to understand causal questions is to know the different stages of causality.
Confounders: A Crucial element in Causality
Let us imagine a scenario where we conduct an experiment to measure the outcome of an event. We will use mortality and the effectiveness of a drug to cure an ailment. And in this experiment there are two groups with people randomly assigned to each group. Group A and Group B with the first group taking a drug and the second group given a placebo. What this means is that there should be no fundamental difference between those in A or in B. Thus any observation found in either of these groups must be because of the experiment itself and not some other factor. That means for us to make an observation, it will be because of the efficacy or otherwise of the drug and not because of some other factor such as allergies or lifestyle. But here lies the problem. If there is another factor which ensures a difference between Group A and Group B, it cannot be conclusive in saying that the difference between the two groups was because of the efficacy of the drug. It means there could be other significant factors or variables which affect the outcome of the result. Factors like some men in Group A are senior citizens or some women in Group B are pregnant. This is a confounder. Understanding how confounders work and their influence on results will take us a step further into understanding causality.
Mediators Are Important to Establish Causality
The discovery of causation is but a tip of the iceberg in understanding causality. The crux is to know why an action causes an outcome. If we can find out why small cars flip over during accidents, it can help us make small cars safer and prevent the rate of accidents. In this scenario, a mediator is a link between an action and an outcome. For example, burglar alarms are prevalent to prevent theft. But the alarm does not detect burglars. What it detects is movement. So if a burglar was to break into an apartment through an attic window, what the alarm detects is the movement of the burglar which sends the signal for the alarm to sound. The mediator between the burglar and the alarm in this scenario is the movement.
Burglar > movement > alarm.
But mediators can misunderstand situations which can lead to poor outcomes. Let us use the alarm situation once again. But this time a car anti-theft alarm. These alarms have sensors which prompts a signal to the alarm when the sensors are breached. Some of these sensors are programmed to trigger the alarm where the window is broken or the door handle is touched. Some are noise-detecting sensors. The problem so happens where a bird lands on your car roof and the alarm sounds. Or the noise from a low-flying aircraft triggers the alarm. Does it mean your car is being stolen? No. This is a simple scenario. But misunderstanding mediators have led to far more serious and fatal consequences. The ability to understand mediators can help us in our journey to establish causality.
By understanding the Known, We Can Predict Or Expect The Unknown
This is how algorithms work. The ability to use set parameters to create a mathematical formula which helps to show the relationship between correlation and causation. And because mathematics is logic, the beneficiaries of this process would be Artificial Intelligence. It could help us understand not only the answers we seek but the uncertainties in that answer. And this is the future.
Key Quotes
Here’re some key quotes from the book:
“Data can tell you that the people who took a medicine recovered faster than those who did not take it, but they can’t tell you why. Maybe those who took the medicine did so because they could afford it and would have recovered just as fast without it.” – Judea Pearl, The Book of Why: The New Science of Cause and Effect.
“If I could sum up the message of this book in one pithy phrase, it would be that you are smarter than your data. Data do not understand causes and effects; humans do.” – Judea Pearl, The Book of Why: The New Science of Cause and Effect.
Conclusion
Understanding the relationship between cause and correlation can be the key to advance mankind and unlock the potential of Artificial Intelligence.
Since You’re Here …
Great books should be read, studied and reviewed, so reading the actual book may provide more value to you than the book insights on this page. This will also support the work of the book author and what we do on LarnEdu.
Furthermore, you can support LarnEdu by making a one-off or monthly donation (via PayPal) for as little as £0.99 or by sharing this content.
Got any feedback or suggestions? Use the comment section below or send us a message.
Content created by: Chima